General Finance
Nov 20, 2024
•
5 min read
Top 4 Challenges Working with Unstructured Data in Financial Services
The Importance of Unstructured Data in Financial Services
We recently had the opportunity to dive into the role of unstructured data in financial services and it’s immense growth in recent years - fueled by digital transformation, increasing customer engagement across digital channels, and regulatory pressures. In fact, it’s estimated that over 80% of data in financial services is unstructured, ranging from emails and call transcripts to social media data and legal documents.
This data holds valuable information for decision-making, risk assessment, and customer engagement, but it’s often underutilized due to how difficult it is to process. Most financial institutions that manage to leverage this data, will tend to have fully staffed teams (e.g. data science, machine learning, etc.) and access to advanced tooling - making this process less than ideal for the masses.
Today we’ll explore the top four challenges that financial institutions face when dealing with unstructured data and we’ll even introduce a new and innovative way that will help make the job a lot easier.
Top 4 Challenges Working with Unstructured Data
1) Data Integration and Fragmentation
Financial institutions face considerable hurdles in integrating unstructured data, which originates from a wide array of sources such as emails, customer service transcripts, social media, legal contracts, and news reports. Each source uses varied formats—text, audio, video—and integrating these into a cohesive system is complex and resource-intensive. Additionally, data silos within organizations make it difficult to create a unified view, causing data fragmentation that limits visibility and insight. Take a look at how this may play out within different disciplines in financial services.
Banking: In banking, data integration is critical for building a comprehensive view of customers across different departments. Integrating customer service transcripts, credit reports, and social media insights helps banks offer personalized services and detect potential issues before they escalate.
Brokerages: Brokerages need to integrate news feeds, analyst reports, and market sentiment data for effective trading strategies. Fragmentation in data makes it difficult for traders to have a cohesive view of market conditions, impacting decision-making in real-time.
Wealth Management: Wealth managers rely on integrating customer emails, financial statements, and legal documents to provide holistic advice. Data fragmentation can hinder their ability to provide tailored investment solutions that are needed to maximize ROI.
If you’re working with unstructured data, unifying data is essential for making smarter decisions, improving operational efficiency, and enhancing customer experiences.
2) Data Privacy and Compliance
Unstructured data in financial services often contains sensitive information such as personal identifiers, transaction details, and customer communication logs. This makes data privacy and compliance a significant challenge, especially in an industry heavily regulated by standards like GDPR, FINRA, and the SEC. Managing unstructured data while ensuring compliance requires a robust governance framework to prevent data breaches and unauthorized access.
Unlike structured data, which can be uniformly encrypted and managed, unstructured data can be a bit more challenging. That means that for every data type from audio transcripts to emails, must meet compliance standards.
Payments: In the payments sector, unstructured data from transaction notes and customer support interactions must comply with PCI-DSS and GDPR. Ensuring that this data is properly encrypted and accessible only by authorized personnel is crucial to avoid penalties.
Banking: Banks face challenges in ensuring that customer call transcripts and email communications comply with regulatory requirements. For instance, the storage and retrieval of these records must meet strict standards set by regulatory bodies.
Brokerages: Brokerages handling client communication logs must ensure that all communications are archived and easily retrievable for compliance with SEC regulations, adding to the complexity of managing unstructured data.
3) Data Quality and Noise
Unstructured data often lacks consistency and quality, which complicates efforts to derive actionable insights. For instance, customer feedback in emails may contain irrelevant information, and sentiment analysis of social media posts may misinterpret sarcasm or nuances, leading to inaccurate insights. Financial services must filter out “noise” and standardize data to maintain quality and reliability.
Achieving high data quality from unstructured sources demands advanced data cleaning and normalization processes. However leveraging large language models are useful for extracting relevant information, but require fine-tuning to handle industry-specific terms and interpret complex language accurately. Without rigorous data quality control, unstructured data can lead to incomplete or misleading conclusions, impacting decision-making and customer experiences.
Wealth Management: Wealth managers must extract meaningful insights from client communications, which often contain unstructured feedback. Poor data quality can lead to misinterpretation of client needs and inappropriate investment advice.
Transaction Cost Analysis (TCA) in Trading: In trading, analyzing unstructured market data and trader notes requires high data quality. Inaccurate data can lead to erroneous conclusions in transaction cost analysis, impacting profitability.
Corporate Actions in Asset Management: Processing corporate actions involves extracting information from various documents, such as press releases and shareholder reports. The unstructured nature of these documents makes it challenging to maintain data quality, which is essential for accurate portfolio management.
4) Resource-Intensive Processing
Extracting insights from unstructured data is resource-intensive, requiring advanced AI, machine learning, and natural language processing technologies. Financial institutions often face a lack of in-house expertise and infrastructure capable of processing large-scale unstructured data efficiently. This is particularly challenging as financial data needs to be processed in near real-time to detect fraud, assess risk, or respond to market trends.
Investing in the right technology stack, skilled personnel, and processing power is essential for deriving value from unstructured data. Some financial services adopt AI-driven solutions that automate parts of the process, but these solutions still require specialized expertise for deployment and maintenance. Without the proper resources, unstructured data remains an untapped asset that is costly to process and difficult to operationalize.
Fraud Detection in Payments: Real-time fraud detection requires the processing of unstructured transaction notes and customer interactions. Without sufficient processing power and expertise, identifying fraudulent patterns becomes difficult.
Risk Assessment in Insurance: Insurance companies need to analyze unstructured data from claim notes, medical reports, and customer calls to assess risk accurately. The resource-intensive nature of this analysis can delay decision-making, affecting customer satisfaction.
Trade Surveillance in Brokerages: Brokerages must analyze trading communications, such as emails and chat transcripts, to detect market manipulation. Processing this unstructured data in real-time is crucial but requires significant computational resources and specialized expertise.
Unlocking Your Unstructured Data
As the volume of unstructured data in financial services continues to grow, so does the opportunity to automate processes and drive meaningful improvements in patient care and operational efficiency. Today, most financial institutions and fintech companies rely on their teams to manually process the data in order to get around data formatting and structure. However, this process is both labor-intensive and susceptible to errors due to the sheer amount of volume and variability in the data. To solve this, Gradient developed a new way for businesses to interact with data - providing the first and only AI-powered Data Reasoning Platform that enables businesses to forge both structured and unstructured data to create data workflows that were unimaginable with traditional tools.
Gradient’s Data Reasoning Platform
Gradient’s Data Reasoning Platform is the first AI-powered and SOC 2 Type 2 compliant platform that’s designed to automate and transform how financial institutions and fintech companies handle their most complex data workflows. Powered by a suite of proprietary large language models (LLMs) and AI tools, Gradient eliminates the need for manual data preparation, intermediate processing steps, or a dedicated ML team to maximize the ROI from your data. Unlike traditional data processing tools, Gradient’s Data Reasoning Platform doesn’t require teams to create complex workflows from scratch and manually tune every aspect of the pipeline.
Schemaless Experience: The Gradient Platform provides a flexible approach to data by removing traditional constraints and the need for structured input data. Enterprise organizations can now leverage data in different shapes, formats, and variations without the need to prepare and standardize the data beforehand.
Deeper Insights, Less Overhead: Automating complex data workflows with higher order operations has never been easier. Gradient’s Data Reasoning Platform removes the need for dedicated ML teams, by leveraging AI to take in raw or unstructured data to intelligently infer relationships, derive new data, and handle knowledge-based operations with ease.
Continuous Learning and Accuracy: Gradient’s Platform implements a continuous learning process to improve accuracy that involves real-time human feedback through the Gradient Control System (GCS). Using GCS, enterprise businesses have the ability to provide direct feedback to help tune and align the AI system to expected outputs.
Reliability You Can Trust: Precision and reliability are fundamental for automation, especially when you’re dealing with complex data workflows. The Gradient Monitoring System (GMS) identifies anomalies that may occur to ensure workflows are consistent or corrected if needed.
Designed to Scale: Typically the more disparate data you have, the bigger the team you’ll need to process, interpret, and identify key insights that are needed to execute high level tasks. Gradient enables you to process 10x the data at 10x the speed without the need for a dedicated team or additional resourcing.
Even with limited, unstructured or incomplete datasets, the Gradient Data Reasoning Platform can intelligently infer relationships, generate derived data, and handle knowledge-based operations - making this a completely unique experience. This means that teams can automate even the most intricate workflows at the highest level of accuracy and speed - freeing up valuable time and overhead.
Under the Hood: What Makes it Possible
The magic of the Gradient Data Reasoning Platform is its high accuracy, quick time to value, and easy integration into existing enterprise systems.
Data Extraction Agent: Our Extraction Agent intelligently ingests and parses any type of data into Gradient without hassle, including raw and unstructured data. Whether you’re working with PDFs or PNGs we’ve got you covered.
Data Forge: This is the heart of the Gradient Platform. AI automatically reasons about your data - re-shaping, modifying, combining, and reconciling your structured and unstructured data via higher order operations to achieve your objective. Our Data Forge leverages advanced agentic AI techniques to guide the models through multi-hop reasoning reliably and accurately.
Integration Agent: When your data is ready, Gradient will ensure that your data can be easily integrated back into your downstream applications via a simple API.
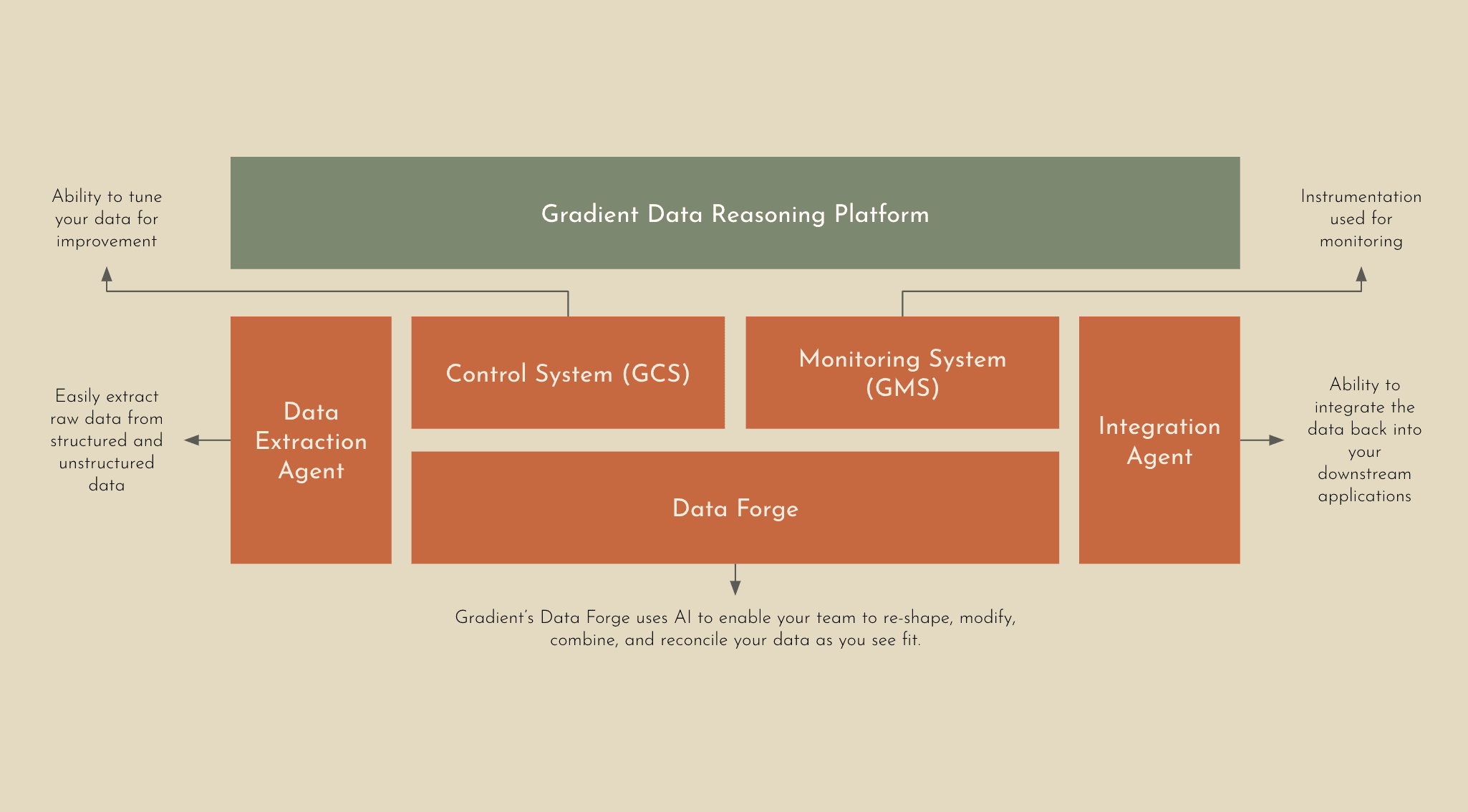
With Gradient, businesses can focus on the outcomes—whether it’s driving customer insights, ensuring regulatory compliance, or optimizing production lines—without getting bogged down in the operational intricacies of data workflows. By automating complex data workflows, organizations can achieve faster, more accurate results at scale - reducing costs and enhancing operational efficiency. In a world where data complexity continues to grow, the ability to harness that data through automation is not just a competitive advantage—it’s a necessity. Take a look at some financial services use cases in detail that financial institutions and fintech companies are using Gradient for today.
Share